Friday, November 11, 2022
Synopsis
The data revolution of the past decade, together with the recent advances in the areas of AI and computation, has a profound social and economic impact. This revolution is changing the way organizations and markets operate. This workshop will draw together leading scholars in the fields of computer science and economics to discuss and debate on the challenges in the era of data economics.
Speakers
About the Series
The IDEAL workshop series brings in experts on topics related to the foundations of data science to present their perspective and research on a common theme. Chicago area researchers with an interest in the foundations of data science. The workshop is part of the IDEAL Fall 2022 Special Quarter on Data Economics.
This workshop is organized by Nicholas Lambert (Mass. Institute of Tech), Annie Liang (Northwestern Univ.), and Haifeng Xu (UChicago).
Logistics
- Date: Friday, November 11, 2022
- In-person: Northwestern University Kellogg Global Hub 5101
- Virtual: via gather.town (details provided to registered participants)
- Registration: click here to register
Transportation and parking
Schedule (tentative)
- 10:30-11:10: Breakfast and Coffee
- 11:10-11:15: Opening Remarks
- 11:15-11:55: Jon Kleinberg (Talk) The Challenge of Understanding What Users Want: Inconsistent Preferences and Engagement Optimization
- 11:55- 12:00: Jon Kleinberg (Q&A)
- 12:00-2:00: Lunch Break
- 2:00-2:40: Alessandro Bonatti (Talk) Data, Competition, and Digital Platforms
- 2:40-2:45: Alessandro Bonatti (Q&A)
- 2:45 – 3:25: Erik Madsen (Talk) Insider Imitation
- 3:25 – 3:30: Erik Madsen (Q&A)
- 3:30 – 4:00: Coffee Break
- 4:00 – 4:40: Rachel Cummings (Talk) The Privacy Elasticity of Behavior: Conceptualization and Application
- 4:40 – 4:45: Rachel Cummings (Q&A)
Titles and Abstracts
Speaker: Jon Kleinberg
Title: The Challenge of Understanding What Users Want: Inconsistent Preferences and Engagement Optimization
Abstract: Online platforms have a wealth of data, run countless experiments and use industrial-scale algorithms to optimize user experience. Despite this, many users seem to regret the time they spend on these platforms. One possible explanation is that incentives are misaligned: platforms are not optimizing for user happiness. We suggest the problem runs deeper, transcending the specific incentives of any particular platform, and instead stems from a mistaken foundational assumption. To understand what users want, platforms look at what users do. This is a kind of revealed-preference assumption that is ubiquitous in user models. Yet research has demonstrated, and personal experience affirms, that we often make choices in the moment that are inconsistent with what we actually want: we can choose mindlessly or myopically, behaviors that feel entirely familiar on online platforms.
In this work, we develop a model of media consumption where users have inconsistent preferences. We consider what happens when a platform that simply wants to maximize user utility is only able to observe behavioral data in the form of user engagement. Our framework is based on a stochastic model of user behavior, in which users are guided by two conflicting sets of preferences — one that operates impulsively in the moment, and the other of which makes plans over longer time-scales. By linking the behavior of this model to abstractions of platform design choices, we can develop a theoretical framework and vocabulary in which to explore interactions between design, behavioral science, and social media.
The talk is based on joint work with Sendhil Mullainathan and Manish Raghavan.
Speaker: Alessandro Bonatti
Title: Data, Competition, and Digital Platforms
Abstract: We propose a model of intermediated digital markets where data and heterogeneity in tastes and products are deÖning features. A monopolist platform uses superior data to match consumers and multiproduct advertisers. Consumers have heterogenous preferences for the advertisersíproduct lines and shop on- or o§-platform. The platform monetizes its data by selling targeted advertising space that allows advertisers to tailor their products to each consumerís preferences. We derive the equilibrium product lines and advertising prices. We identify search costs and informational advantages as two sources of the platformís bargaining power. We show that privacy-enhancing data-governance rules, such as those corresponding to federated learning, can lead to welfare gains for the consumers.
Speaker: Erik Madsen
Title: Insider Imitation
Abstract: We study how regulating data usage impacts innovation in digital markets. Platforms commonly use proprietary data about third-party sellers to inform their own competing offerings, dampening incentives for innovation. We model this interaction and characterize how data usage restrictions reshape these incentives. We apply our results to show that a ban on data usage has an ambiguous impact on innovation dictated by the thickness of the right tail of demand for new products. We then generalize our analysis to more flexible regulations controlling when and what data is made available, showing how each of these additional levers should be used to improve the effectiveness of regulation. Our results contribute to an ongoing policy discussion regarding competition in digital markets.
Speaker: Rachel Cummings
Title: The Privacy Elasticity of Behavior: Conceptualization and Application
Abstract: We propose and initiate the study of privacy elasticity—the responsiveness of economic variables to small changes in the level of privacy given to participants in an economic system. Individuals rarely experience either full privacy or a complete lack of privacy; we propose to use differential privacy—a computer-science theory increasingly adopted by industry and government—as a standardized means of quantifying continuous privacy changes. The resulting privacy measure implies a privacy-elasticity notion that is portable and comparable across contexts. We demonstrate the feasibility of this approach by estimating the privacy elasticity of public-good contributions in a lab experiment.
(joint work with Inbal Dekel, Katrina Ligett, and Ori Heffetz)
Paper available here: https://papers.ssrn.com/sol3/papers.cfm?abstract_id=4145498
Join Our Newsletter
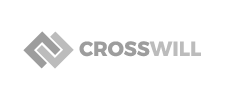