Wednesday, June 7th, 2023
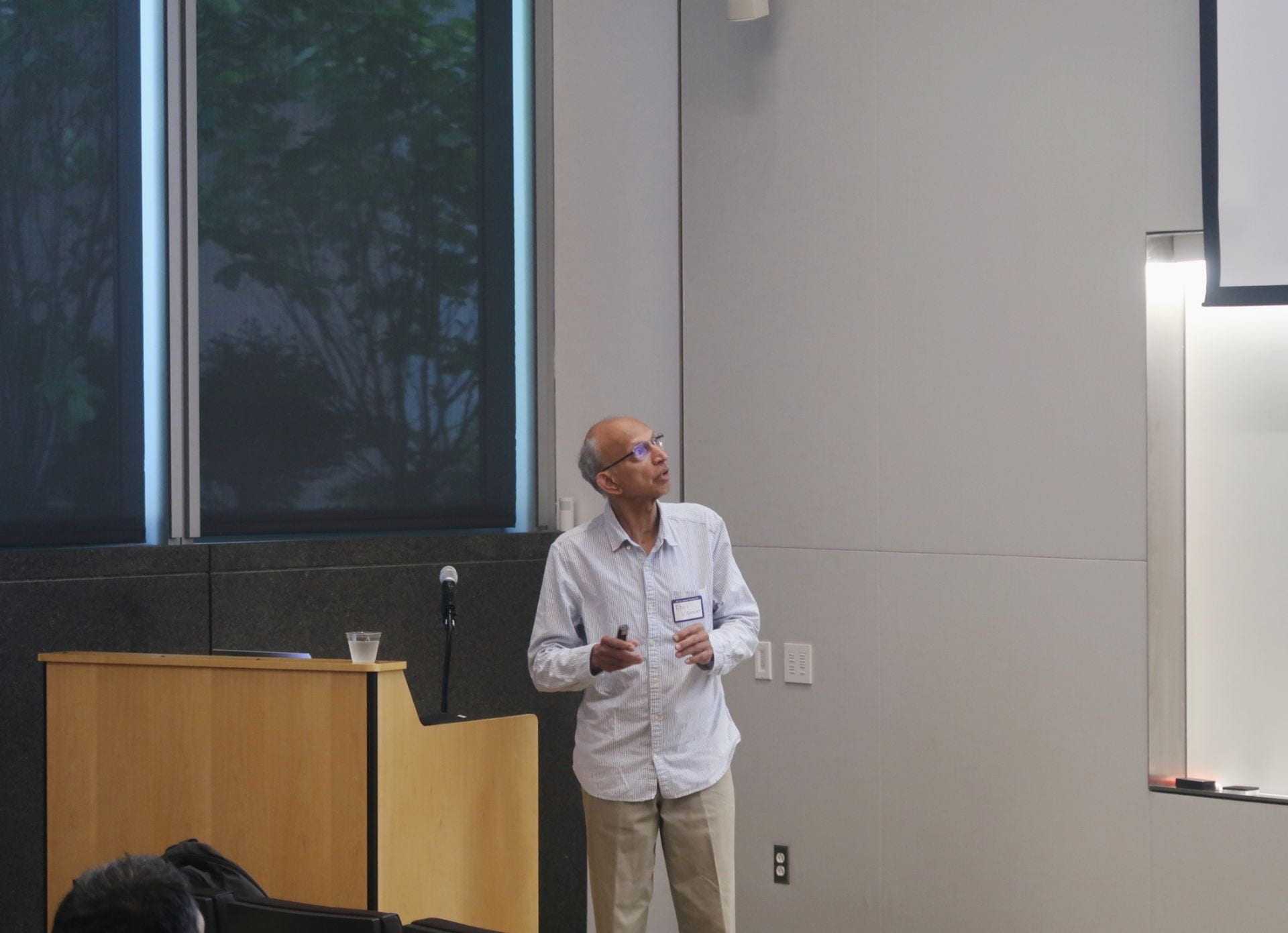
Ravi Kannan
Logistics
- Date: Wednesday, June 7th, 2023
- Location: Simpson-Querrey Auditorium, Northwestern Simpson Querrey Biomedical Research Center (303 E Superior St, 1st floor)
- Registration: click here to register

Alekh Agarwal
Schedule
9:00 am – 9:30 am | Opening remarks, leadership updates from Avrim Blum, Lev Reyzin, Aravindan Vijayaraghavan, Will Perkins |
9:30 am – 10:15 am | Keynote: Ravi Kannan Data, Geometry and Algorithms |
10:15 am – 10:30 am | Break |
10:30 am – 11:15 am | Keynote: Alekh Agarwal Optimistic Posterior Sampling: A General Purpose Reinforcement Learning Solution? |
11:15 am – 12:00 pm |
Thrust 1 Foundations of Machine Learning technical program (chaired by Natasha Devroye) Lightning talks: Avrim Blum: Strategic Classification
Natasha Devroye: Interpreting deep learned error correcting codes
Rad Niazadeh: Multi-scale online learning
Lev Reyzin: Query Learning and Littlestone Dimension
Chun Liu: Energetic variational inference in machine learning
Milos Zefran: Physical data and inference for systems interacting with the physical world |
12:00 pm – 1:00 pm | Working lunch + coffee |
1:00 pm – 2:30 pm |
Thrust 2 High-Dimensional Data Analysis and Inference technical program (chaired by Antonio Auffinger and Yichao Wu) 1:00-1:25pm Reza Gheissari High-dimensional limit theorems for stochastic gradient descent |
2:30 pm – 2:45 pm | Break |
2:45 pm – 4:15 pm |
Thrust 3 Data Science and Society technical program (chaired by Ivan Canay) 2:45-3:15pm Eric Auerbach Heterogeneous treatment effects for networks, panels, and other outcome matrices. Lightning talks: Vijay Kamble: Incentives for Exploration in Markets (Economics + Learning interface) |
4:15 pm – 6:00 pm | Student poster session with refreshments |

Avrim Blum

Lev Reyzin

Natasha Devroye

Milos Zefran

Titles and Abstracts
Speaker: Reza Gheissari (Northwestern University)
Title: High-dimensional limit theorems for stochastic gradient descent
Abstract: Stochastic gradient descent (SGD) is the go-to method for large-scale optimization problems in modern data science. Often, these settings are data constrained, so the sample size and the dimension of the parameter space have to scale together. In this “high-dimensional” scaling, we study pathwise limits of the SGD. Namely, we show limit theorems for trajectories of reasonable summary statistics (finite-dimensional functions of the SGD) as the dimension goes to infinity and the step size simultaneously goes to zero. The limits that arise can be complicated ODE’s or SDE’s, depending on the initialization, step-size and space rescaling. We present these general results, and then discuss their implications for some concrete tasks including classification of XOR Gaussian mixtures via two layer networks. Based on joint work with Gerard Ben Arous and Aukosh Jagannath.
Speaker: Cong Ma (UChicago)
Title: Optimally tackling covariate shift in RKHS-based nonparametric regression
Abstract: We study the covariate shift problem in the context of nonparametric regression over a reproducing kernel Hilbert space (RKHS). We focus on two natural families of covariate shift problems defined using the likelihood ratios between the source and target distributions. When the likelihood ratios are uniformly bounded, we prove that the kernel ridge regression (KRR) estimator with a carefully chosen regularization parameter is minimax rate-optimal (up to a log factor) for a large family of RKHSs with regular kernel eigenvalues. Interestingly, KRR does not require full knowledge of the likelihood ratio apart from an upper bound on it. In striking contrast to the standard statistical setting without covariate shift, we also demonstrate that a naive estimator, which minimizes the empirical risk over the function class, is strictly suboptimal under covariate shift as compared to KRR. We then address the larger class of covariate shift problems where the likelihood ratio is possibly unbounded yet has a finite second moment. Here, we propose a reweighted KRR estimator that weights samples based on a careful truncation of the likelihood ratios. Again, we are able to show that this estimator is minimax optimal, up to logarithmic factors.
Speaker: Yuri Makarychev (TTIC)
Title: Higher-Order Cheeger Inequality for Partitioning with Buffers
Abstract: We prove a new generalization of the higher-order Cheeger inequality for partitioning with buffers. Consider a graph G. The buffered expansion of a set of vertices S with a buffer B is the edge expansion of S after removing all the edges from S to its buffer B. An ε-buffered k-partitioning of graph G is a partitioning of G into disjoint components P_1, …, P_k and buffers B_1, …, B_k in which the size of each buffer B_i is at most ε|P_i|. We prove that there exists an ε-buffered k-partitioning of G, in which the buffered expansion of each P_i with buffer B_i is at most O_𝛿((log k) / ε) λ_{k’}, where where λ_{k’} is the k’-th smallest eigenvalue of the normalized Laplacian of G and k’ = (1 + 𝛿) k.
Our inequality is constructive and avoids the “square-root loss” that is present in the standard Cheeger inequalities (even for k=2). We also provide a complementary lower bound, and a generalization to the setting with arbitrary vertex weights and edge costs. Moreover our result implies and generalizes the standard higher-order Cheeger inequalities and another recent Cheeger-type inequality by Kwok, Lau, and Lee (2017) involving robust vertex expansion.
Joint work with Konstantin Makarychev, Liren Shan, and Aravindan Vijayaraghavan


Lunch & Break
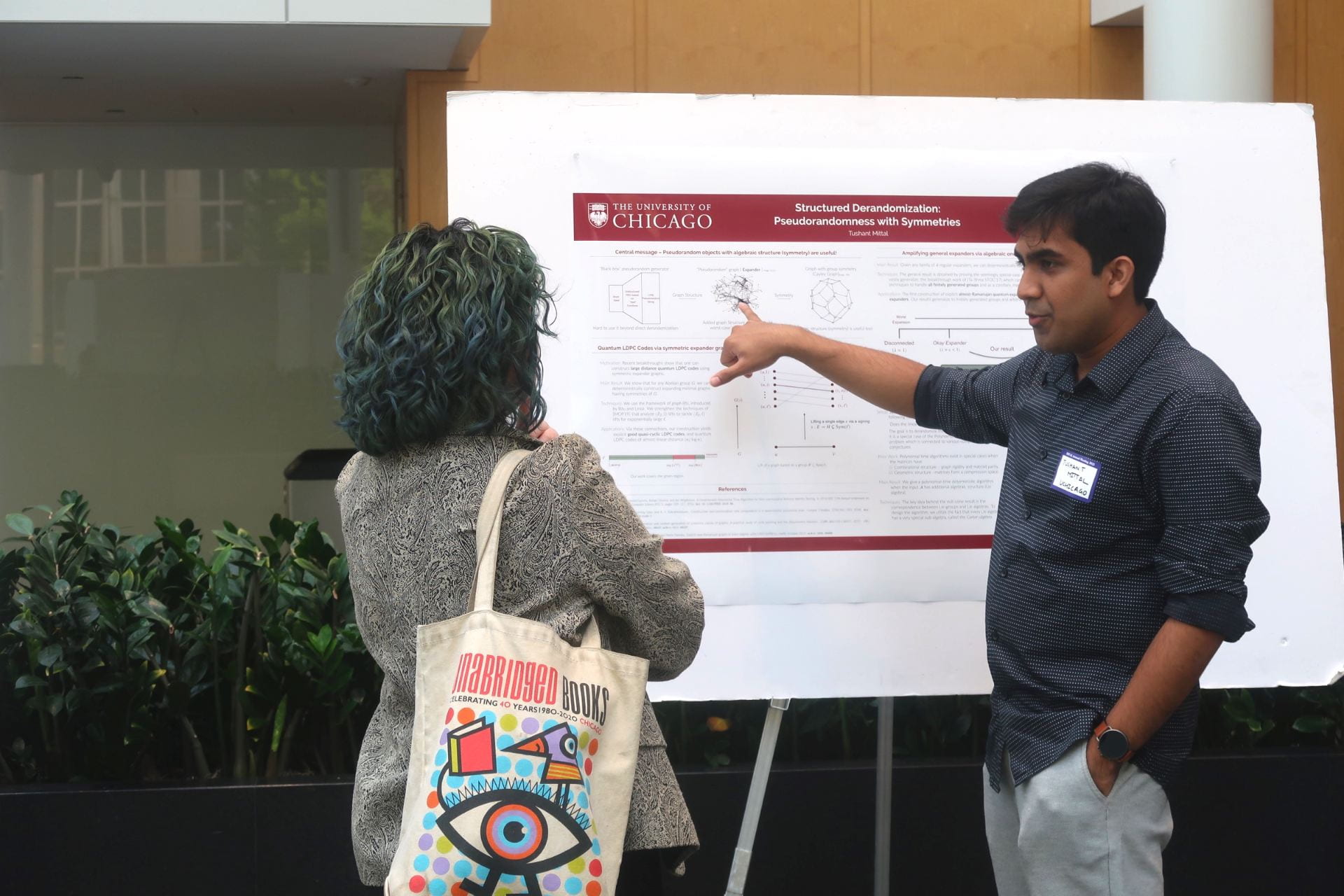





Poster Session
Poster List
All IDEAL-supported students and postdocs are expected to present a poster during the poster session. Others are welcome to present posters if we have the space. The poster should be able to fit on a 40in x 60in foam board. We will provide foam boards, stands and pins, but you must bring the poster!
Shishir Adhikari (UIC): Inferring causal effects under heterogeneous peer influence.
Zahra Fatemi (UIC): Contagion Effect Estimation Using Proximal Embeddings
Yifan Guo (UChicago): An Isotonic Mechanism for Overlapping Ownership
Minbiao Han (UChicago): First-order Convex Fitting and Its Application to Economics and Optimization
Anmol Kabra (TTI-C): Modeling Strategic Behavior in Hospital Rating Systems.
Tim Tsz-Kit Lau (Northwestern): Global Optimization of Nonsmooth Weakly Convex Functions via Simulated Annealing.
Gene Li (TTI-C): When is Agnostic Reinforcement Learning Statistically Tractable?
Tushant Mittal (UChicago): Structured Derandomization: Beyond black-box pseudorandomness
Abhijeet Mulgund (UIC), Raj Shekhar (UIC): Interpreting deep-learned error correcting codes
Zohreh Ovaisi (UIC): Fairness of Interaction in Ranking under Exposure, Selection, and Trust Bias
Pawan Poojary (Northwestern): Bayesian learning in Mean-Field Games with observational noise, authored by Pawan Poojary and Dr. Randall Berry.
Liren Shan (Northwestern): Random Cuts are Optimal for Explainable k-Medians.
Shubham Singh (UIC): Fair Resource Allocation with Heterogeneous Agents.
Vaidehi Srinivas (Northwestern): The Burer-Monteiro SDP method can fail even above the Barvinok-Pataki bound
Jacob Thomas (IIT): Chicago Water Crisis: Statistical Analysis of Testing Practices
Fan Yao (UVA/UChicago): How Bad is top-K Recommendation under Competing Content Creators?
Percy Zhai (UChicago): Gaussian Approximation of Covariance for High-Dimensional Long-Memory Time Series
More details are forthcoming..
Parking
Parking Garages Nearby:
Erie Ontario
321 East Erie Street
Huron Superior
222 East Huron Street